Case Study - Maximizing Sales Efficiency: AI-Powered Lead Prioritization
Learn how AI-powered lead scoring and prioritization can transform your sales operations, leading to higher conversion rates and more efficient resource allocation.
- Client
- Retail
- Year
- Service
- ML Model Development, Integration & Deployment
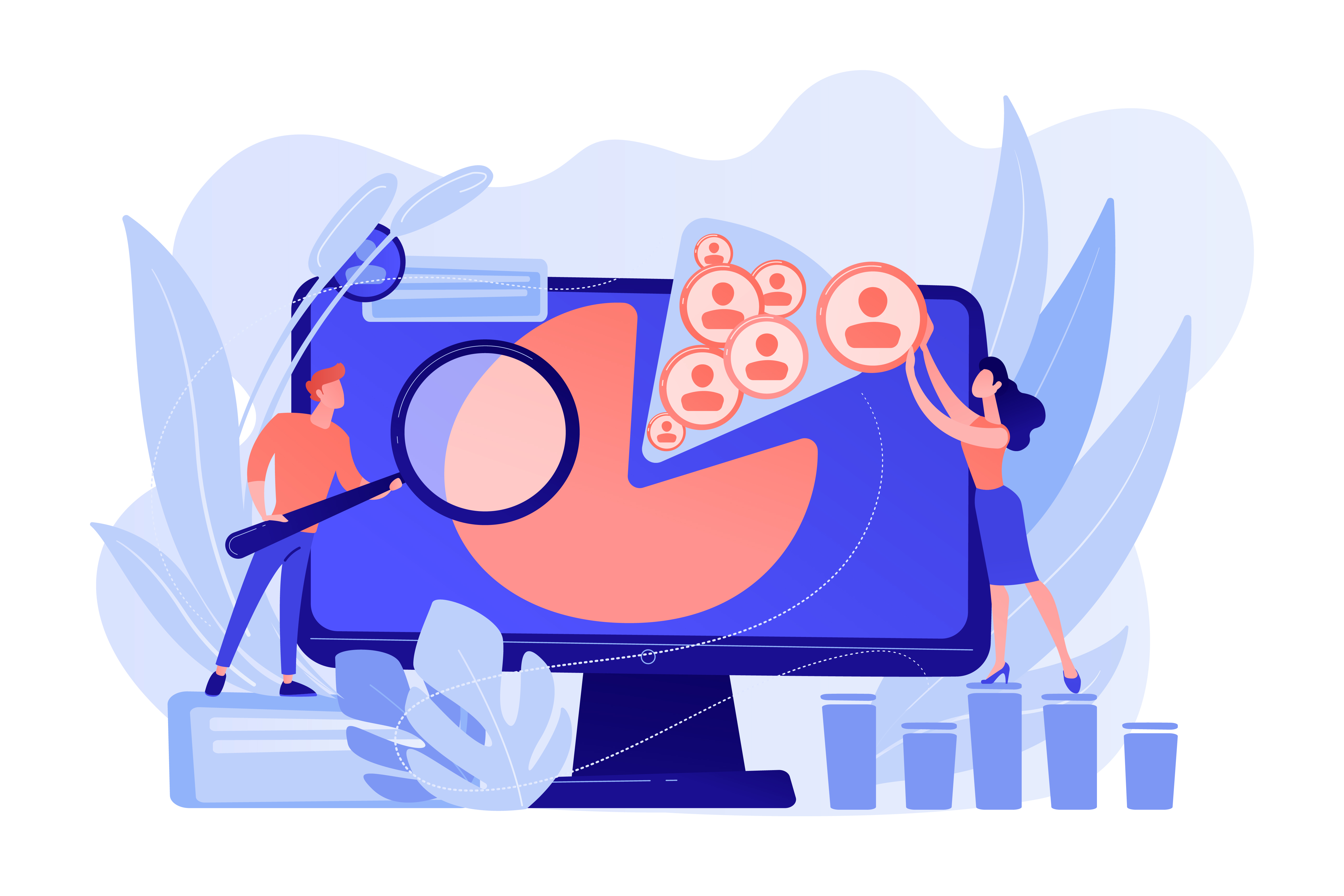
The Challenge
Sales teams at large companies face a universal challenge: managing an overwhelming number of potential leads with limited time and resources. Our retail client was struggling with exactly this problem - their sales team was managing thousands of potential leads monthly, with no reliable way to determine which ones deserved immediate attention.
Their sales pipeline suffered from several key pain points:
Inefficient prioritization
Sales representatives were manually reviewing hundreds of leads weekly based primarily on intuition and basic criteria like time since last outreachInconsistent approach
Different team members used different methods to decide which leads to pursue firstLengthy onboarding
New sales team members required months of training to develop effective prioritization skillsData silos
Valuable information existed across multiple systems but wasn't being connected in meaningful ways
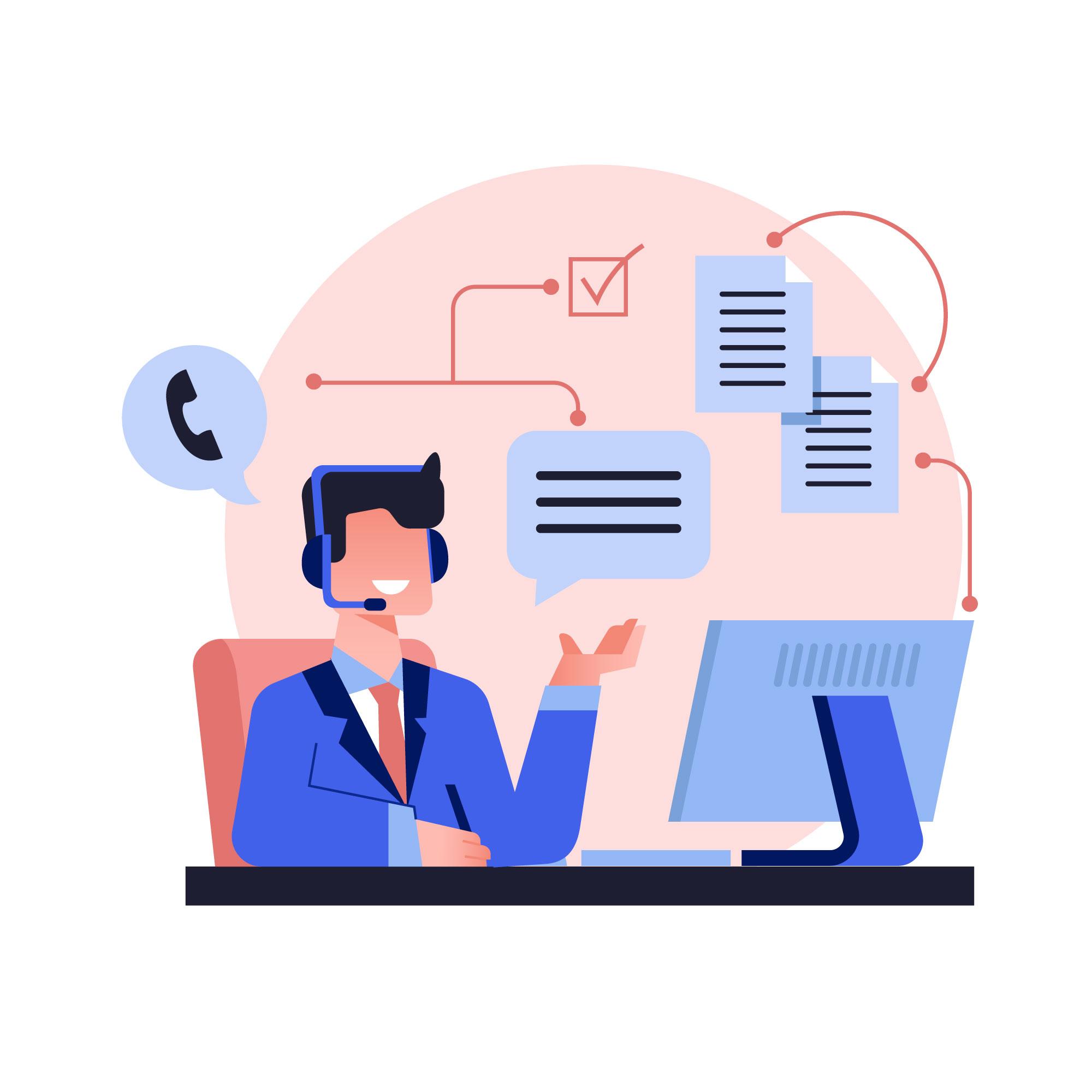
Our Solution: A Data-Driven Approach
Rather than replacing their existing CRM system, we designed an AI-powered lead scoring solution that integrated with their current workflows while dramatically improving prioritization effectiveness.
Phase 1: Unifying Fragmented Data
Connecting data that had always existed in separate parts of the organization ended up being a game changer for our client.
Customer demographics
Integrating customer information including census data, loyalty program tier, and geographic locationHistorical patterns
Analyzing past purchasing behavior and product interestsDigital engagement
Incorporating website visits, email interactions, and content interacted withSales interactions
Logging call history, meeting notes, and proposal statusMarket context
Adding seasonal factors and industry-specific timing considerations
Phase 2: Building a Custom Prediction Engine
AI Lead Scoring Pipeline
Our end-to-end machine learning pipeline transforms fragmented data into actionable lead scores
Data Sources
Input data collection
Unified data from CRM, marketing data, website interactions, sales activities, and external industry data
Feature Engineering
Data transformation
Converting raw data into meaningful features for the AI model, including temporal patterns and interactions
ML Models
Prediction engine
Ensemble of gradient boosting models trained on historical conversion data to predict lead quality
Explainability Component
Transparency
SHAP values and feature importance analysis to explain predictions in business-friendly terms to build trust in the model
Integration
User interface
Our pipeline integrated into the company event-driven architecture, delivering scored leads directly into CRM systems with explanations and recommendations
Key Technical Features
A/B Testing
Designed a rigorous experiment setup with carefully designed treatment and control groups of sales representatives to empirically validate that our system outperformed the previous manual processScalable Architecture
Inference pipeline deployed to Vertex AI in GCP, providing scalable batch processing with automatic resource allocation and optimizationMonitoring & Alerting
Real-time data drift detection and performance monitoring using GCP Cloud Monitoring with automated alerts for model degradationContinuous Learning
Leveraged our monitoring system to trigger training pipelines for new models, which are evaluated online by competing with the champion model, splitting traffic in an A/B testing framework
Phase 3: Seamless Workflow Integration
CRM integration
Delivering prioritized leads directly in their existing system with minimal disruptionTransparent scoring
Providing clear explanations for why specific leads received high scoresTiming recommendations
Suggesting optimal moments for follow-up based on engagement patternsTalking points
Offering data-driven conversation starters based on lead characteristicsMinimal retraining
Designing the interface to match existing workflows, reducing adoption friction
Results: Measurable Business Impact
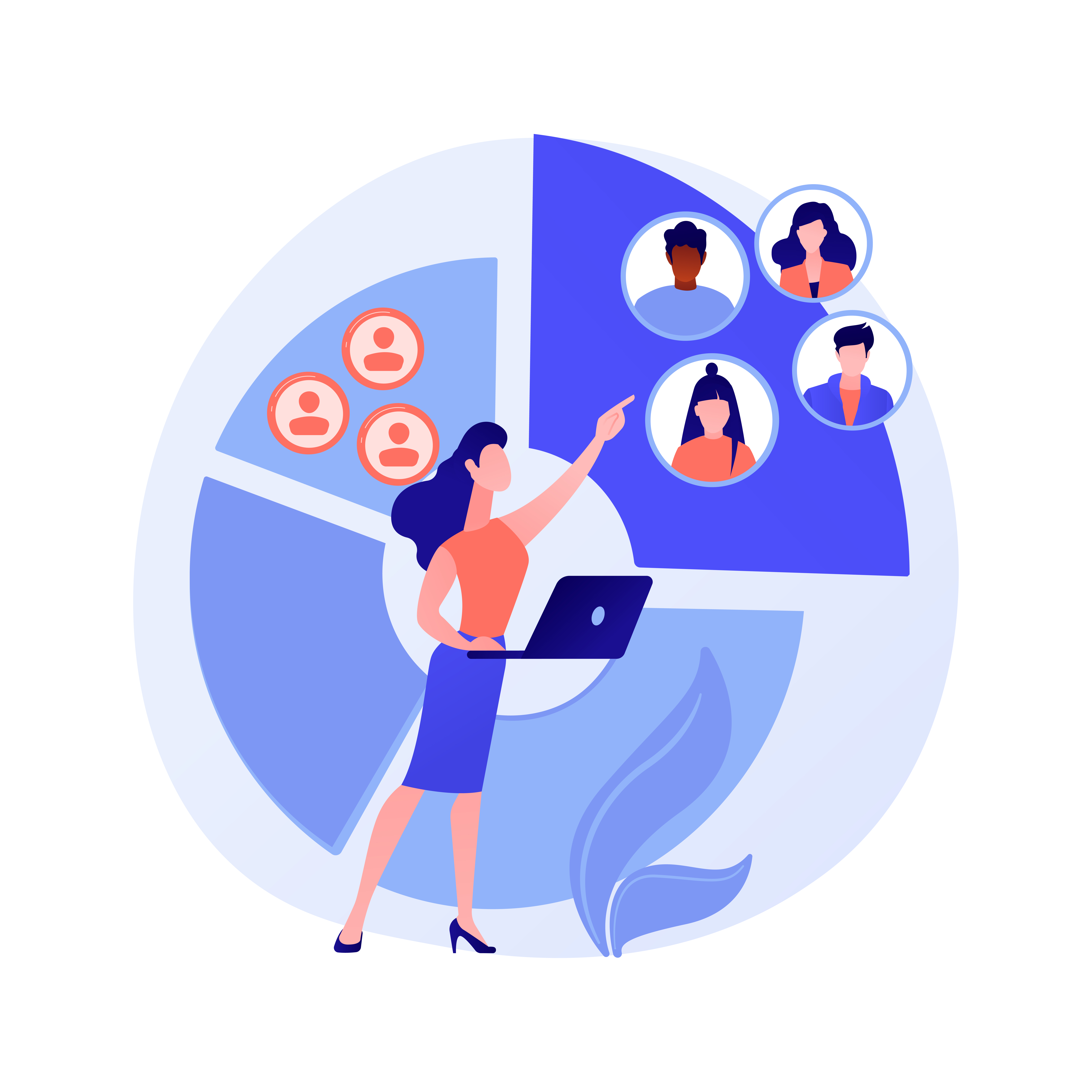
- Increase in conversion rate
- 40%
- Reduction in sales cycle length
- 28%
Sales Funnel Comparison
Conversion Rate by Stage (%)
Starting from 100% of initial contacts, showing conversion rates through the sales funnel
Key Insights:
- AI-powered prioritization shows significantly higher conversion rates at the last stage
- Initial stages are similar, ensuring no extra resources are needed
What's particularly notable is how the system redistributed sales efforts without requiring additional resources. By focusing attention on high-probability leads at the right moments, the team achieved significantly better outcomes without increasing headcount.
Implementation Insights
Start with a focused use case
We began with the enterprise sales team, allowing us to deliver value quickly and gather feedback from a motivated stakeholder group before broader deploymentInvest in change management
Technical solutions require human adoption, so we included workshops, side-by-side performance tracking, and continuous feedback loopsEmbrace transparency
Sales professionals need to understand why the system makes recommendations, so we prioritized explainable AI approachesMeasure what matters
We established clear baseline metrics before implementation and tracked progress against specific business objectives
The Path Forward
AI-Powered Roadmap
The initial implementation was just the beginning. Together with the client, we established a roadmap for ongoing enhancement:
Expansion
Extending to additional sales teams and channels with customized models tailored to specific product lines and market segments
Data Enrichment
Incorporating new data sources as they become available for improved accuracy and more nuanced lead qualification metrics
Advanced Features
Testing more sophisticated recommendation features based on emerging patterns and real-time engagement signals
Marketing Integration
Building deeper connections with marketing automation systems for unified customer journey optimization
Transform Your Sales Operations
Every organization's sales process is unique, but the fundamental challenge is universal: how to allocate limited resources for maximum impact. AI-powered lead prioritization offers a proven approach to solving this challenge.
- Higher conversion rates
- More efficient resource allocation
- Enhanced sales team productivity
- Data-driven decision making
Don't let your most promising opportunities slip through the cracks. We can help you harness the power of AI to transform your approach to lead prioritization and help your sales team achieve their full potential.